Minimizing GPU Cost for Your Deep Learning on Kubernetes
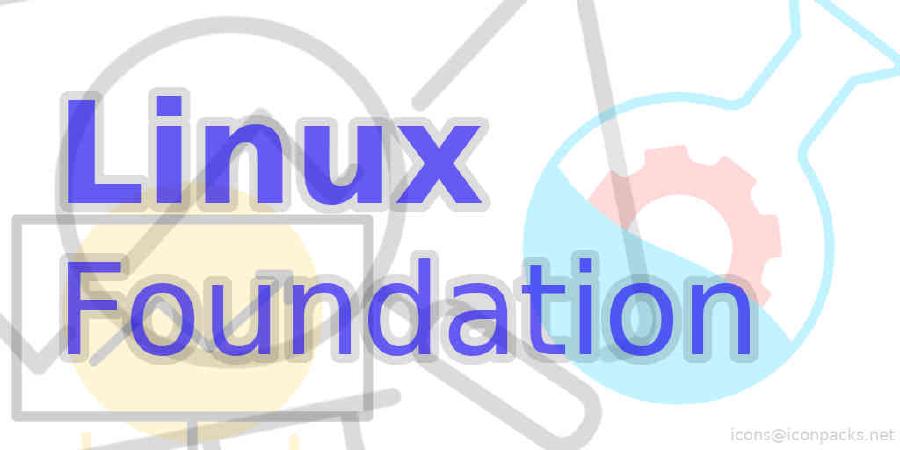
More and more data scientists run their Nvidia GPU based deep learning tasks on Kubernetes. Meanwhile, it's found over 40% cost are wasted on idle GPU in the cluster. So one important challenge is how …
Talk Title | Minimizing GPU Cost for Your Deep Learning on Kubernetes |
Speakers | Kai Zhang (Staff Engineer, Alibaba), Yang Che (Senior Engineer, Alibaba) |
Conference | KubeCon + CloudNativeCon |
Conf Tag | |
Location | Shanghai, China |
Date | Jun 23-26, 2019 |
URL | Talk Page |
Slides | Talk Slides |
Video | |
More and more data scientists run their Nvidia GPU based deep learning tasks on Kubernetes. Meanwhile, it’s found over 40% cost are wasted on idle GPU in the cluster. So one important challenge is how Kubernetes can help to improve GPU usage efficiency. In this talk we will introduce a GPU sharing solution on native Kubernetes. All design and implementation details will be discussed. Key topics include, - How to define GPU sharing API - How to make GPU sharing can be scheduled in the Kubernetes cluster without changing scheduler bare bone code. - How to integrate GPU isolation solution with Kubernetes A demo will be shown to illustrate how Tensorflow users to run different jobs on the same GPU device in Kubernetes cluster. In practise of the solution , overall GPU usage gets remarkable improvement, especially for AI model develop, debug and inference services.