Economics and Best Practices of Running AI/ML Workloads on Kubernetes
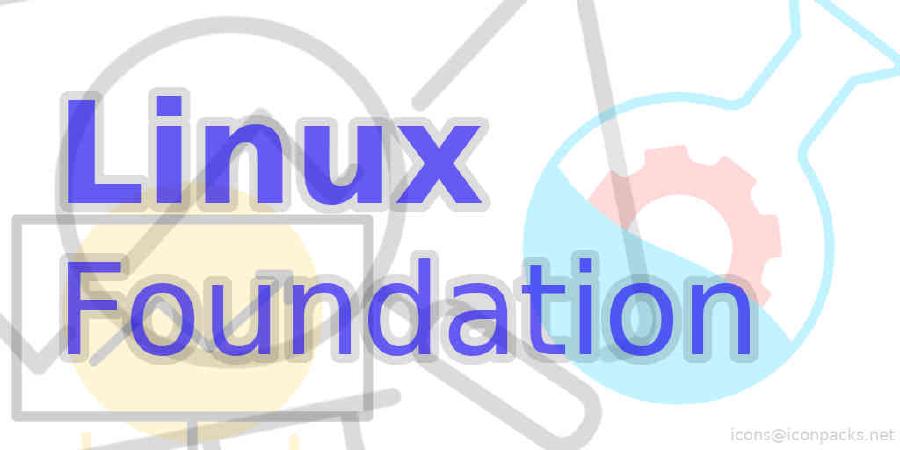
In this session, we will discuss how Kubernetes driven AI/ML building blocks are making AI/ML simple, fast and efficient for data scientists, data engineers, devops engineers and everyday users. We wi …
Talk Title | Economics and Best Practices of Running AI/ML Workloads on Kubernetes |
Speakers | Yaron Haviv (CTO, Iguazio), Maulin Patel (Product Manager, Google) |
Conference | KubeCon + CloudNativeCon Europe |
Conf Tag | |
Location | Barcelona, Spain |
Date | May 19-23, 2019 |
URL | Talk Page |
Slides | Talk Slides |
Video | |
In this session, we will discuss how Kubernetes driven AI/ML building blocks are making AI/ML simple, fast and efficient for data scientists, data engineers, devops engineers and everyday users. We will explore how Kubernetes, Kubeflow and Kubeflow pipeline can help to mitigate complexities and challenges associated with AI/ML. We will demonstrate the use of Accelerators like GPUs and TPU in Kubernetes Engine to make serving compute intensive ML/AI workloads easy, fast and scalable. We will present the real world examples of commonly used AI/ML applications, discuss their performance and share best practices. We will also present how the economics are different when it comes to ML workloads and highlight the unique values Kubernetes brings to enterprises.